Hi, I'm Falicon. I build stuff.
My real name is Kevin Marshall and I'm a husband, dad, startup geek, software developer, youth sports coach, sometimes early stage investor, and all-the-time gamer.
I like building, learning, & creating things so I'm almost always involved in multiple things at once. I put this site together to serve as a bit of mashup of those various interests, projects, and whatever my latest distractions might be.
I hope you'll find at least a nugget or two of something useful & interesting here. And, as always, if you've got questions or just want to chat about something please email me at kevin at falicon.com any time!
Follow my stream
I've been live streaming some old school gaming sessions lately — so if you're into that sort of thing, I would love to have you check it out and follow my channel.
Consider becoming an insider
If you're interested in behind-the-scenes access to everything I have going on including some exclusive games, content, and apps then you should become an insider.
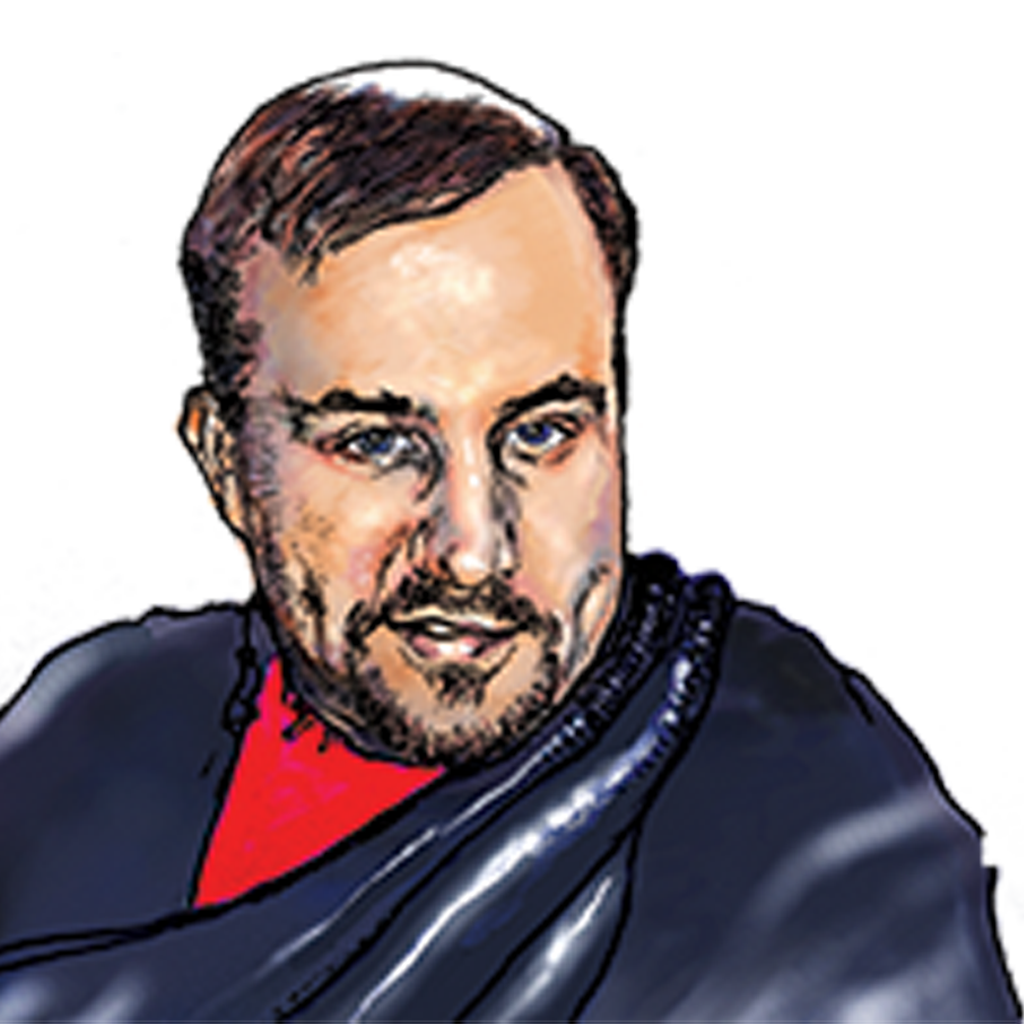
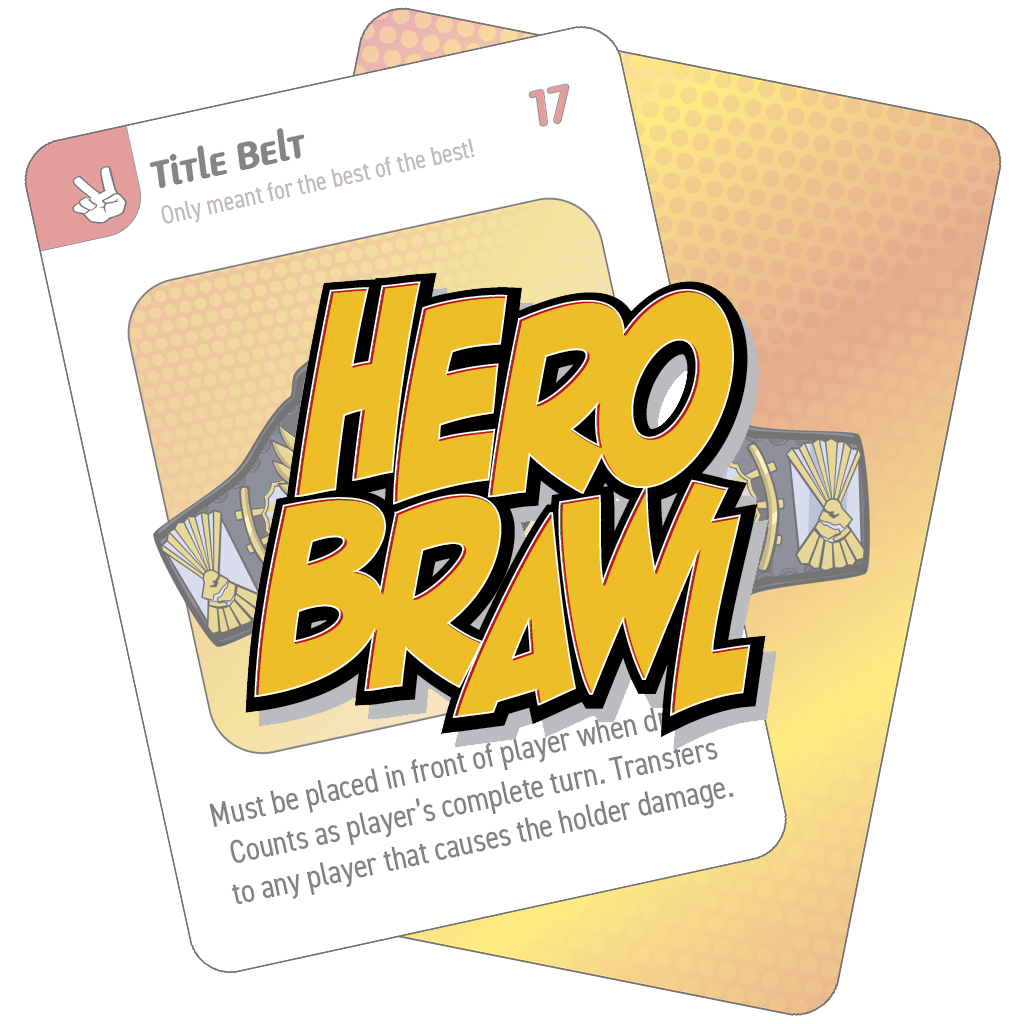
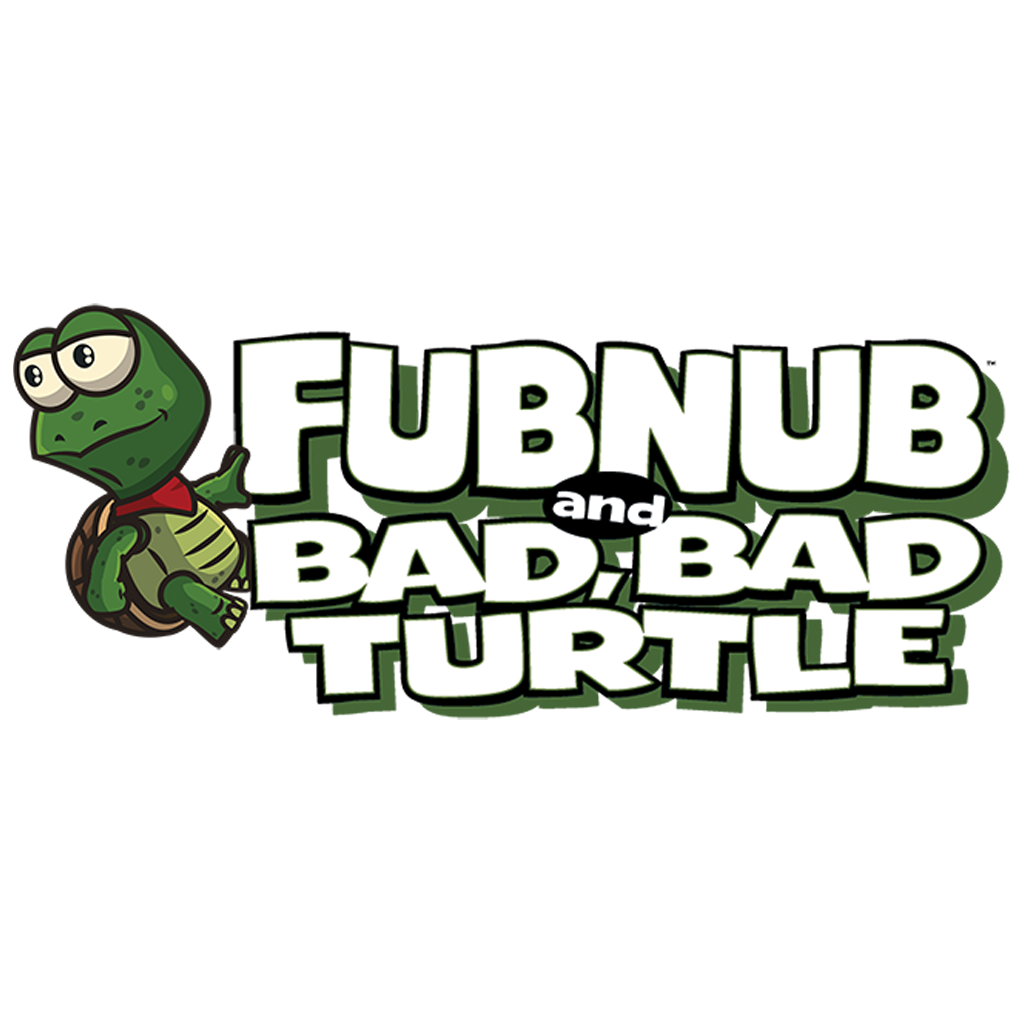
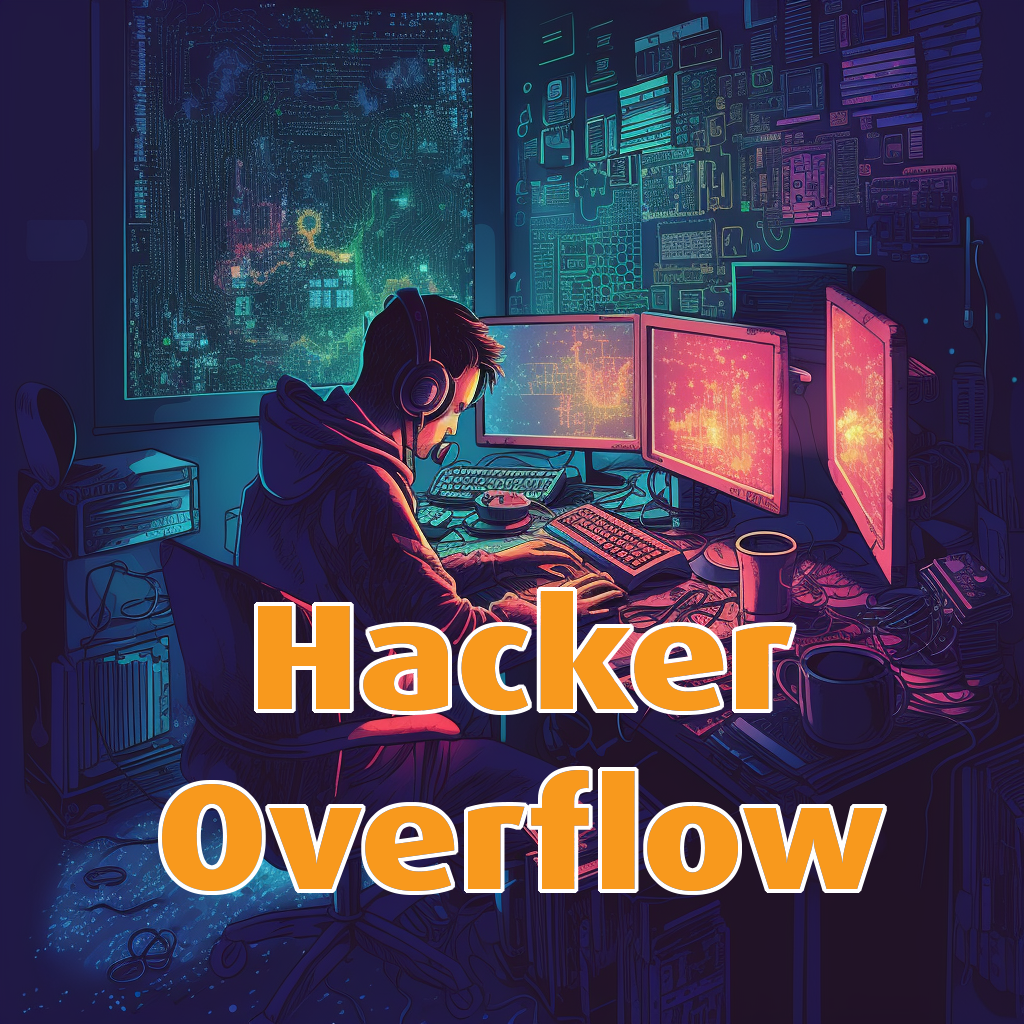
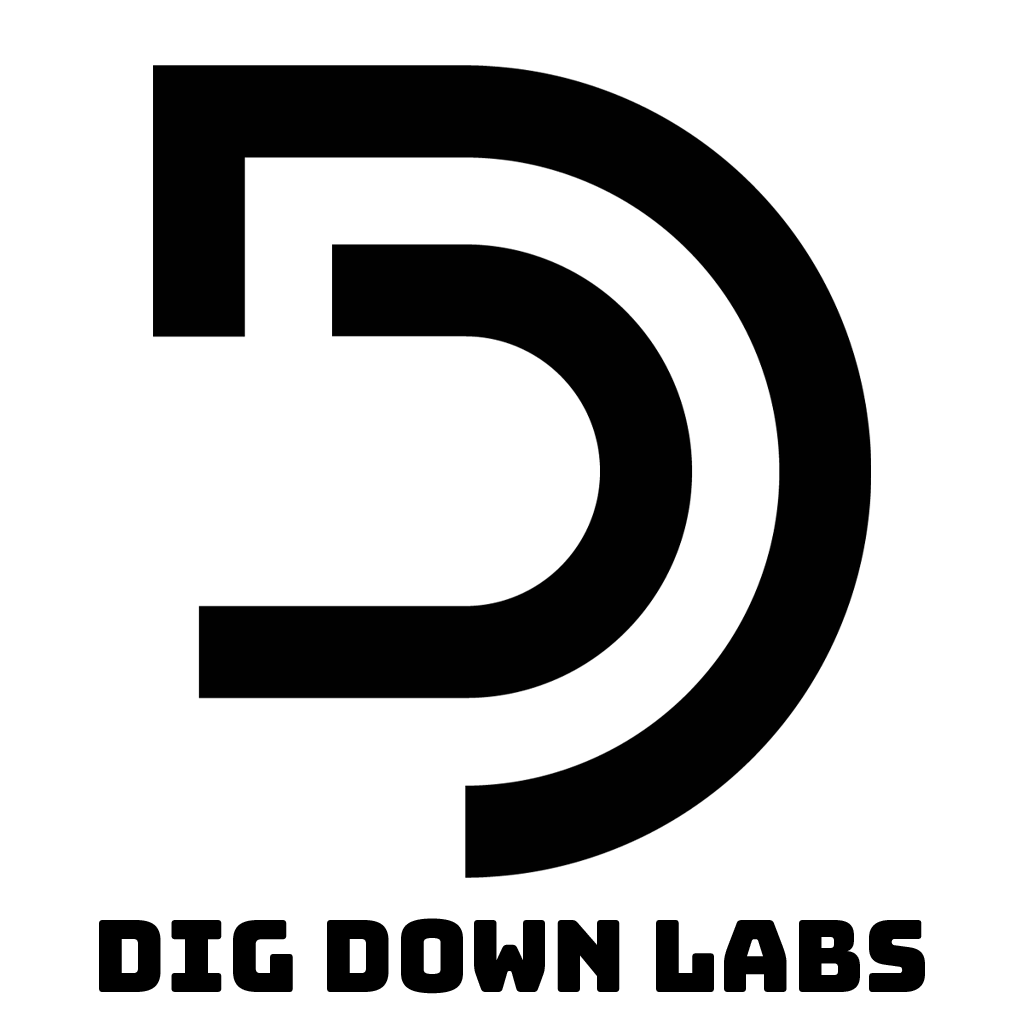